Artificial Intelligence (AI)- vol:7
Back <<
Robotic Personal Assistants:
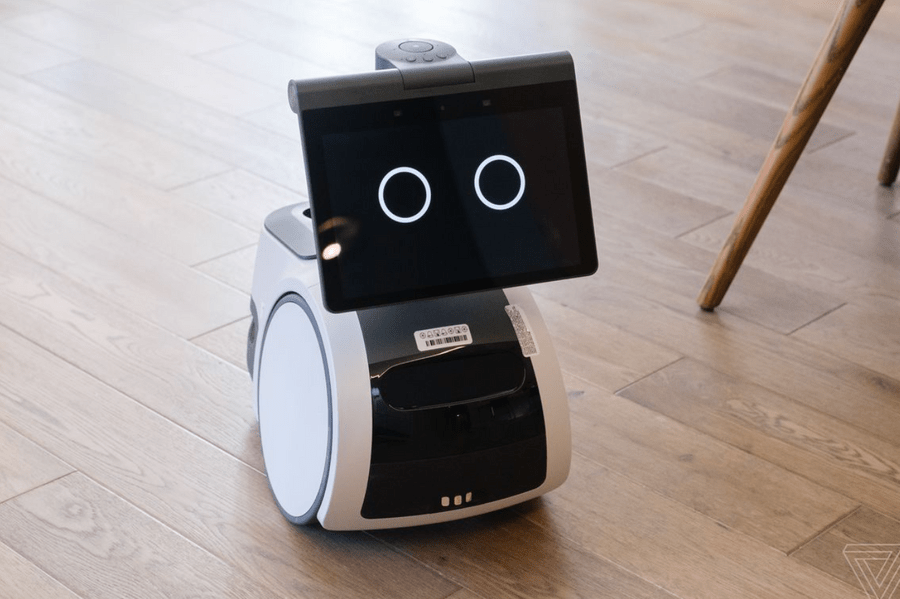
Introduction:
In an increasingly fast-paced and interconnected world, the demand for efficient and personalized assistance has never been greater. Robotic personal assistants have emerged as a technological solution to this need, seamlessly integrating into our daily lives to simplify tasks, enhance productivity, and provide companionship. This article explores the growing prominence of robotic personal assistants, their capabilities, and their impact on reshaping our interactions with technology.
Defining Robotic Personal Assistants:
Robotic personal assistants are advanced machines equipped with artificial intelligence (AI) and natural language processing capabilities. These intelligent machines are designed to understand and respond to human commands, perform tasks autonomously, and adapt to users’ preferences over time. Unlike traditional virtual assistants confined to software interfaces, robotic personal assistants combine physical embodiment with digital intelligence, enabling them to interact with the physical world.
Capabilities and Features:
- Voice Interaction: Robotic personal assistants rely on sophisticated speech recognition technology to understand spoken commands and queries. They can engage in natural language conversations, execute tasks, and provide information and recommendations based on user inquiries.
- Task Automation: These assistants excel at automating routine tasks, such as setting reminders, managing calendars, sending messages, and controlling smart home devices. They streamline daily activities, saving time and effort for users.
- Personalization: Robotic personal assistants leverage AI algorithms to learn users’ preferences, adapt to their behavior patterns, and provide personalized recommendations. Whether it’s suggesting music based on listening habits or offering tailored news updates, these assistants strive to enhance the user experience.
- Environmental Awareness: Equipped with sensors and cameras, robotic personal assistants can perceive and interact with their surroundings. They navigate spaces autonomously, avoiding obstacles and can perform physical tasks like fetching objects or cleaning.
- Emotional Engagement: Some robotic personal assistants are designed to recognize and respond to human emotions. They incorporate facial recognition and emotion-detection algorithms to provide empathetic and personalized interactions, fostering a sense of companionship.
Applications in Daily Life:
Robotic personal assistants are finding their way into various aspects of daily life, transforming the way we live and work.
- Smart Homes: Integrating with smart home devices, robotic personal assistants act as central hubs, enabling seamless control of lighting, temperature, security systems, and entertainment devices through voice commands.
- Elderly Care: With an aging population, robotic personal assistants are crucial in elderly care. They can monitor vital signs, provide medication reminders, assist with daily tasks, and offer companionship, improving the quality of life for older adults.
- Education and Learning: Robotic personal assistants are utilized in educational settings to support interactive learning experiences. They can answer questions, explain, and adapt their teaching methods to individual students, enhancing engagement and knowledge retention.
- Customer Service: Businesses are deploying robotic personal assistants in customer service roles to handle inquiries, provide product recommendations, and offer support. These assistants can improve customer satisfaction and streamline service processes.
Challenges and Future Developments:
While robotic personal assistants hold tremendous potential, several challenges need to be addressed for their widespread adoption.
- Privacy and Security: As these assistants collect vast amounts of personal data, ensuring data privacy and implementing robust security measures are essential to building user trust.
- Ethical Considerations: The development of robotic personal assistants raises ethical questions, such as the potential for bias in decision-making algorithms or the impact on human employment. Addressing these concerns is crucial to ensure responsible and equitable use of the technology.
- Seamless Integration: Future advancements will focus on seamlessly integrating robotic personal assistants into various domains, from healthcare to transportation, to provide comprehensive support and services.
- Enhanced Intelligence: Further developments in AI and machine learning will enable robotic personal assistants to possess
Artificial Intelligence (AI)- vol:6
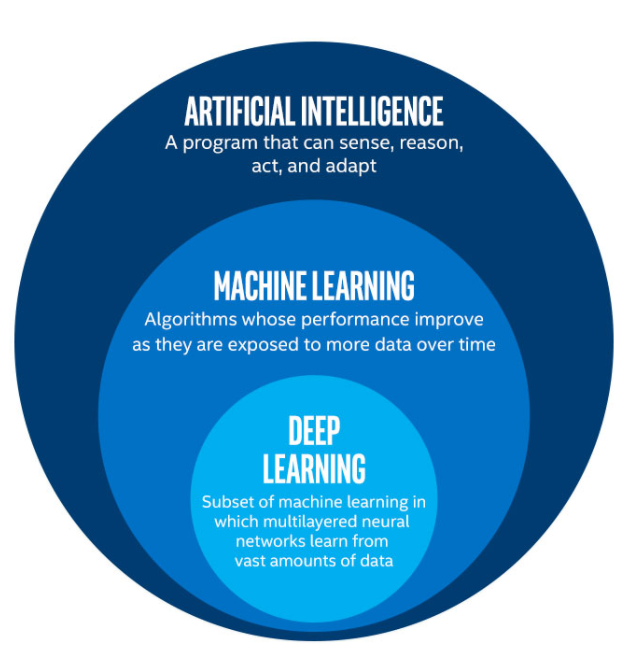
Introduction:
In recent years deep learning has emerged as a transformative force in the field of artificial intelligence. By mimicking the human brain’s neural networks, deep learning algorithms have revolutionized various domains, including computer vision, natural language processing, speech recognition and more. This article explores the essence of deep learning, its applications and its impact on shaping the future of AI.
Understanding Deep Learning:
Deep learning is a subset of machine learning that leverages artificial neural networks to process and learn from vast amounts of data. The key differentiator of deep learning is its ability to automatically discover and extract meaningful patterns and features from raw data, eliminating the need for manual feature engineering.
At the core of deep learning lies the artificial neural network, inspired by the complex interconnected structure of the human brain. Neural networks consist of multiple layers of interconnected nodes, known as neurons. Which process and transform data as it flows through the network. Each neuron applies a mathematical operation to its inputs and passes the result to the next layer, ultimately producing an output.
Training Deep Learning Models:
Training a deep learning model involves two fundamental steps: forward propagation and backward propagation. During forward propagation, input data passes through the layers of the neural network, and the network computes predictions. These predictions are then compared to the desired outputs, and an error or loss function quantifies the disparity between them.
Backward propagation, also known as backpropagation, is the process by which the network adjusts its internal parameters to minimize the error. It calculates the gradients of the error with respect to the model’s parameters and uses optimization algorithms, such as stochastic gradient descent, to update the parameters iteratively. This iterative optimization process allows the model to learn and improve its predictions over time.
Applications of Deep Learning:
Deep learning has demonstrated remarkable performance across a wide range of applications, fueling advancements in various industries.
- Computer Vision: Deep learning has revolutionized computer vision tasks, such as object detection, image recognition, and image segmentation. Convolutional neural networks (CNNs) excel at learning hierarchical representations from images, enabling breakthroughs in autonomous driving, medical imaging, and surveillance systems.
- Natural Language Processing (NLP): Deep learning techniques have significantly improved language-related tasks, including sentiment analysis, machine translation, and text generation. Recurrent neural networks (RNNs) and transformer models, such as the Transformer architecture, have achieved state-of-the-art results in language understanding and generation.
- Speech Recognition: Deep learning algorithms, particularly recurrent neural networks and their variants, have greatly enhanced automatic speech recognition systems. Voice assistants, transcription services and voice-controlled applications owe their effectiveness to these advancements.
- Healthcare: Deep learning has made significant strides in medical image analysis, enabling early detection of diseases like cancer, aiding in diagnosis, and improving treatment planning. It has also shown promise in drug discovery, genomics and personalized medicine.
- Autonomous Systems: Deep learning plays a vital role in autonomous systems, such as self-driving cars and drones. These systems rely on deep learning algorithms for perception, decision-making, and control, allowing them to navigate complex environments safely.
The Future of Deep Learning:
As deep learning continues to evolve, its potential impact on society and various industries is immense. Here are a few areas where deep learning is expected to make significant strides.
- Explainability and Interpretability: Deep learning models often act as black boxes, making it challenging to understand their decision-making process. Future research aims to develop methods for explaining and interpreting deep learning models, improving transparency and trustworthiness.
- Transfer Learning and Few-shot Learning: Transfer learning techniques enable the transfer of knowledge learned from one task or domain to another, reducing the need for large amounts of labeled
Cloud Computing (cnt..)
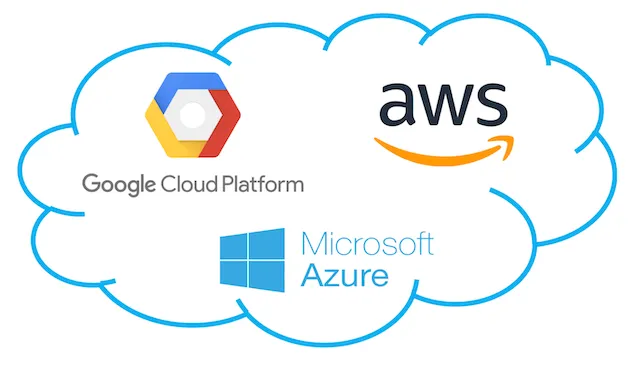
Cloud computing has become an essential part of modern business and personal computing. It is a model for delivering on-demand access to shared computing resources, including servers, storage, applications, and services over the internet.
Cloud computing has revolutionized the way we use technology. Instead of having to rely on expensive, dedicated hardware and software, cloud computing allows us to access the resources we need from any device, anywhere in the world. This makes it possible for businesses to scale up their operations quickly and cost-effectively, without having to invest in expensive infrastructure.
One of the primary benefits of cloud computing is its flexibility. Cloud computing allows businesses to quickly and easily scale their operations up or down as their needs change. For example, a company can quickly provision additional computing resources during peak periods of demand, and then release those resources when the demand subsides. This level of flexibility is simply not possible with traditional on-premise computing solutions.
Another significant benefit of cloud computing is its cost-effectiveness. With cloud computing, businesses only pay for the resources they use, rather than having to invest in and maintain expensive hardware and software. This makes it possible for small and medium-sized businesses to compete with larger enterprises on a level playing field.
Cloud computing also offers enhanced security and reliability. Cloud providers have significant expertise in securing their networks and infrastructure against cyber attacks, and they typically have multiple data centers around the world to ensure high availability and redundancy.
Finally, cloud computing enables collaboration and remote work. By providing employees with access to shared resources and applications, cloud computing makes it easy for teams to collaborate and work together from anywhere in the world.
Overall, cloud computing has become an essential tool for modern business and personal computing. Its flexibility, cost-effectiveness, security, reliability, and collaboration capabilities make it an excellent choice for any organization looking to stay competitive in today’s fast-paced, digital world.
Here are some of the most common types of cloud computing:
- Infrastructure as a Service (IaaS) IaaS provides customers with access to virtualized computing resources over the internet. These resources include servers, storage, and networking components that customers can use to build and deploy their own applications and services.
- Platform as a Service (PaaS) PaaS provides customers with a platform on which they can build, test, and deploy applications without having to worry about the underlying infrastructure. The platform provides tools and services for developers, such as databases, middleware, and operating systems.
- Software as a Service (SaaS) SaaS provides customers with access to software applications over the internet. The applications are hosted and managed by the cloud provider, and customers can access them through a web browser or mobile app. Examples of SaaS applications include email, customer relationship management (CRM), and project management tools.
- Public Cloud Public cloud refers to cloud computing services that are available to the general public over the internet. Public cloud providers offer services on a pay-per-use basis, which makes them a cost-effective option for small businesses and individuals.
- Private Cloud Private cloud refers to cloud computing services that are used exclusively by a single organization. Private clouds can be hosted on-premises or in a third-party data center, and they offer greater control over security and customization than public clouds.
- Hybrid Cloud Hybrid cloud refers to a mix of public and private cloud services. With a hybrid cloud, organizations can leverage the scalability and cost-effectiveness of public cloud services while also maintaining control over sensitive data and applications in a private cloud.
- Multi-Cloud Multi-cloud refers to the use of multiple cloud providers to meet an organization’s computing needs. By using multiple cloud providers, organizations can avoid vendor lock-in and take advantage of the strengths of different cloud providers.
Artificial Intelligence (AI)- vol:5
Cognitive cyber security is a rapidly growing field that focuses on using cognitive technologies such as artificial intelligence (AI), machine learning (ML), and natural language processing (NLP) to enhance cyber security measures. The goal of cognitive cyber security is to improve threat detection, response and prevention by making cyber security systems more intelligent and adaptable.
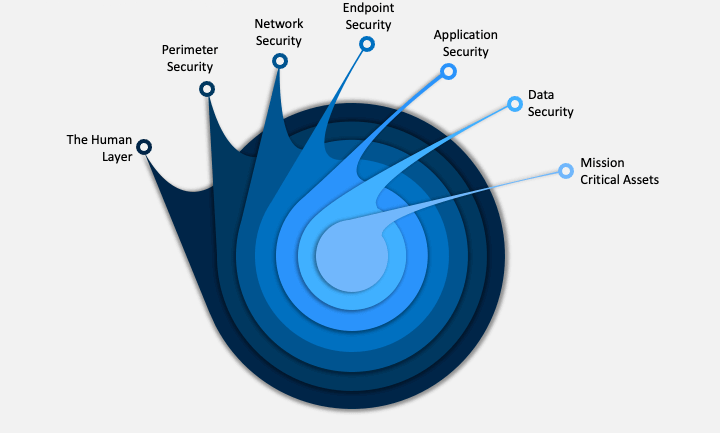
Cognitive cyber security is becoming increasingly important as cyber threats become more sophisticated and frequent. Traditional cyber security measures such as firewalls and antivirus software are no longer sufficient to protect against advanced threats such as “zero day” attacks and social engineering scams. Cognitive cyber security uses advanced technologies to identify these threats and respond to them in real-time.
One of the key advantages of cognitive cyber security is its ability to analyze large volumes of data, quickly and accurately. Cyber security systems can use machine learning algorithms to learn from past attacks and predict future threats. This enables them to detect and respond to threats in real-time before they can cause significant damage.
Another advantage of cognitive cyber security is its ability to adapt to changing threats. Cognitive systems can learn from new data and adjust their responses accordingly. This makes them more effective at detecting and responding to emerging threats, which traditional cyber security measures may not be able to detect.
Cognitive cyber security also has the potential to improve the user experience of cyber security systems. By using natural language processing, cognitive systems can understand user queries and provide relevant information and recommendations. This can help users better understand the risks they face and take appropriate actions to protect themselves.Visit our partners,shoes – leaders in fashionable footwear!
One of the major challenges facing cognitive cyber security is the need for large volumes of high quality data to train machine learning algorithms. This data must also be kept secure to prevent it from falling into the hands of cybercriminals. Additionally, there is a risk that cognitive cyber security systems may become too complex and difficult to manage, leading to errors and false positives.
Despite these challenges, the benefits of cognitive cyber security are clear. By using advanced technologies to enhance threat detection, response and prevention, cognitive cyber security can help organizations protect their valuable assets from cyber threats. As cyber threats continue to evolve, cognitive cyber security will become an essential tool for organizations to stay ahead of the curve and protect themselves from harm.
In conclusion — cognitive cybersecurity is an emerging field that seeks to understand the psychological and behavioral aspects of cybersecurity. By understanding how humans interact with technology and how this interaction can be exploited by cybercriminals, cognitive cybersecurity can help develop strategies to counter cyber threats. This includes educating users about the risks of cyber threats and designing technology that takes into account the way people think and behave. With the growing importance of cybersecurity in our lives, cognitive cybersecurity is likely to play an increasingly important role in protecting against cyber threats in the future.
Digital Engineering
Digital engineering is an emerging field that combines traditional engineering principles with the latest digital technologies to design, simulate and optimize complex systems. This innovative approach to engineering allows engineers to create and test digital models of products and systems before they are physically built, saving time, reducing costs and minimizing errors.
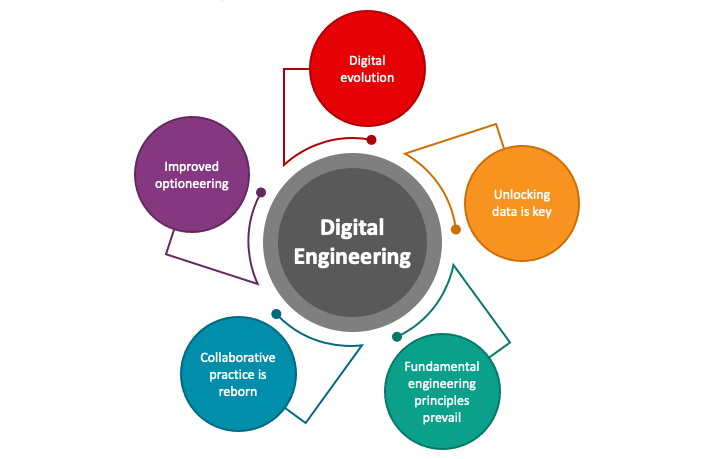
Digital engineering involves the use of computer-aided design (CAD) software to create 2D and 3D models of products and systems. These models can then be used to simulate various scenarios and test the performance of the design in a virtual environment. This approach enables engineers to identify and correct design flaws early in the development process, reducing the need for expensive physical prototypes and iterations.
In addition to CAD software digital engineering also utilizes other advanced technologies such as simulation softwares virtual reality (VR) and augmented reality (AR) systems and cloud computing platforms. Simulation software allows engineers to run complex simulations of products and systems, enabling them to test the performance of the design under different conditions and scenarios. VR and AR systems provide a virtual environment where engineers can visualize and interact with digital models in 3D, making it easier to identify design flaws and make necessary changes. Cloud computing platforms provide a powerful computing infrastructure that can be used to perform complex simulations, analyses and make it possible to test multiple design iterations quickly and efficiently.
One of the major benefits of digital engineering is that it enables engineers to optimize the design of products and systems for performance, reliability and safety. By using advanced simulation tools, engineers can evaluate and compare different design alternatives, and select the one that best meets the requirements of the project. This approach can also help reduce the risk of costly design changes and delays that can occur during the later stages of product development.
Digital engineering is being used in a variety of industries, including aerospace, automotive, energy and construction.
In the aerospace industry — digital engineering is being used to design and test new aircraft and spacecraft, allowing engineers to optimize the design for performance and safety.
In the automotive industry — digital engineering is being used to develop and test new vehicles, including electric and autonomous vehicles.
In the energy industry — digital engineering is being used to design and optimize renewable energy systems such as wind and solar power plants.
In the construction industry — digital engineering is being used to design and simulate complex building systems, such as HVAC and electrical systems.
In conclusion, digital engineering is an exciting and innovative approach to engineering that is transforming the way products and systems are designed and developed. By leveraging advanced technologies such as CAD software, simulation tools, VR and AR systems, and cloud computing platforms, engineers can create and test digital models of products and systems before they are physically built. This approach can help reduce costs, improve performance, and minimize errors, making it an essential tool for engineers in a wide range of industries.
Artificial Intelligence (AI) – vol:4
Machine learning is a type of artificial intelligence, that involves training computers to automatically learn from data and improve over time without being explicitly programmed. The goal of machine learning is to develop algorithms and models that can recognize patterns in data and make predictions/decisions based on that data.
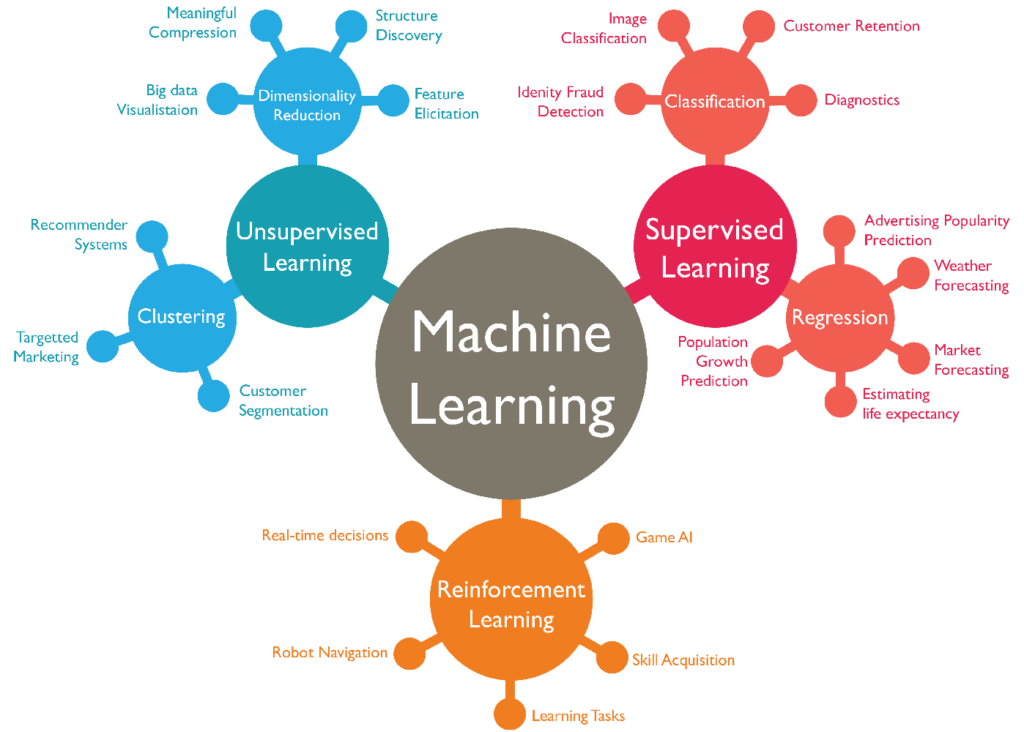
Machine learning is a type of artificial intelligence, that involves training computers to automatically learn from data and improve over time without being explicitly programmed. The goal of machine learning is to develop algorithms and models that can recognize patterns in data and make predictions/decisions based on that data.
Machine learning has become an essential aspect of technology in recent years, with its wide range of applications in various industries such as healthcare, finance and retail. Machine learning is a branch of artificial intelligence that allows computers to learn from data without being explicitly programmed. This capability has revolutionized the way we process, analyze and interpret data.
Machine learning has been applied to many different types of data such as images, text, audio and even video. The algorithms used in machine learning are designed to find patterns and correlations within the data, which allows the computer to make accurate predictions and decisions based on that data.
One of the most commonly used types of machine learning is supervised learning, in this type of learning the computer is provided with a labeled dataset. This means that each data point is already classified into a particular category. A computer uses this labeled dataset to learn the relationship between the input data and the corresponding output, which allows it to predict the output for new input data.
The applications of machine learning are vast and varied. In healthcare, machine learning is used to analyze patient data to identify potential risks and provide personalized treatment plans. In finance — machine learning is used to analyze financial data to identify potential risks and opportunities. In retail — machine learning is used to analyze customer data to provide personalized recommendations and improve the customer experience.
Machine learning has also been applied to natural language processing (NLP) and computer vision. Natural Language Processing allows computers to understand and interpret human language, while computer vision allows computers to interpret and analyze images and video.
Despite the many benefits of machine learning, there are also potential risks and challenges that need to be addressed. One of the main concerns is the potential for bias in the data and algorithms used in machine learning, which could lead to unfair/discriminatory decisions.
In conclusion — machine learning has become an essential aspect of technology with its wide range of applications in various industries. Its ability to learn from data has revolutionized the way we process, analyze and interpret data, and has opened up new opportunities for innovation and growth. However, it is important to address the potential risks and challenges associated with machine learning to ensure that it is used ethically and responsibly.